Modern cutting-edge research operations seem to rely heavily on the ability of GPUs. However, optimized CPUs-based servers have dominated for years because of their computational power and the inherent on-demand price. These processors have represented the driving energy for an assortment of machines including personal computers, public and private clouds, servers, and various types of electronic devices. Over time, CPUs are no longer the superior processor in the market today.
So, what other option is there? The answer is GPUs. The swift evolution of AI (Artificial Intelligence) and amplified difficulty of errands like hosting web platforms and clouds, resulted in the establishment of Graphics Processing Units. GPUs have become significant competitors for CPUs in many areas, especially in data centers and handling AI needs. In this post, we will compare the suitability of data center CPUs and data center GPUs for AI needs.
What is a data center CPU?
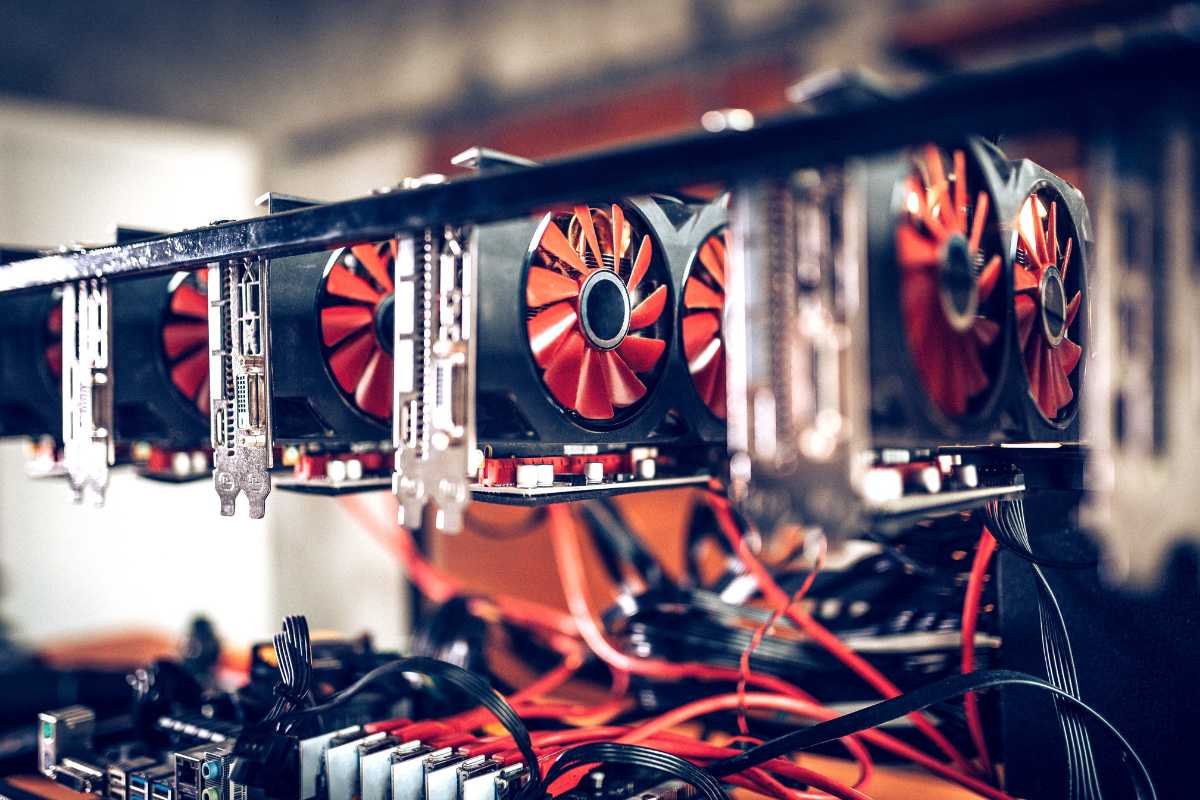
In lame man’s language, a CPU is the brain of a machine, like a computer. However, we can get a bit more technical in this post. The full name is a Central Processing Unit. It is a type of processor used in modern computers. However, it does more than this. It is also applied to data analytic tasks. It was introduced in the tech market in the 1970s. This type of processor consists of multiple cores. It may have between 4 and 64 cores. Each of these cores can execute several instructions in parallel. This makes them versatile for different applications. CPUs emerged for the first time in the 1970s.
What is a data center GPU?
The full name is a Graphics Processing Unit. It is a type of processor that was originally designed for rendering graphics and images. This was its key role when it emerged back in the 1990s. However, since then, people have found ways to increase the applications of GPUs. For example, in data analytics, a GPU is a powerful throttle for tasks. It is made up of thousands of processing cores. These cores can execute tasks in parallel. So, they are suitable for intensive computational tasks.
GPUs and CPUs: Differences in applications
The definitions of CPUs and GPUs touch a bit on their differences when it comes to applications. However, we can clarify these differences even further. One of the key elements of data center GPUs is they can handle parallel deep-learning workloads. This value can be credited to their parallelism. Furthermore, these processors are intended for general purposes. CPUs excel in conducting tasks that need a high single-thread performance. This is thanks of their complex control logic and their large caches. Furthermore, CPUs are great for procedures that need high precision. Let us check out some of the common applications of CPUs:
- Data processing and cleaning in data centers
- Statistical analysis
- Algorithm development and testing
- Indexing and querying
- Management of BI tools (Business Intelligence)
On the other hand, data center XXX CPUs are more versatile when it comes to AI algorithms involving intense memory requirements. They contain thousands of smaller and simpler cores that can achieve parallel processing. This allows data center GPUs to handle multiple tasks simultaneously. GPUs are perfect for operations that can be handled concurrently. Therefore, GPUs are commonly used for the following tasks in data centers:
- Machine and deep learning
- Processing of images and videos
- Visualization of data
- Science-related modeling and simulations
- Mining of cryptocurrencies
So, the key difference is CPUs are ideal for sequential tasks while GPUs are ideal for parallel processing.
GPUs and CPUs: AI applications
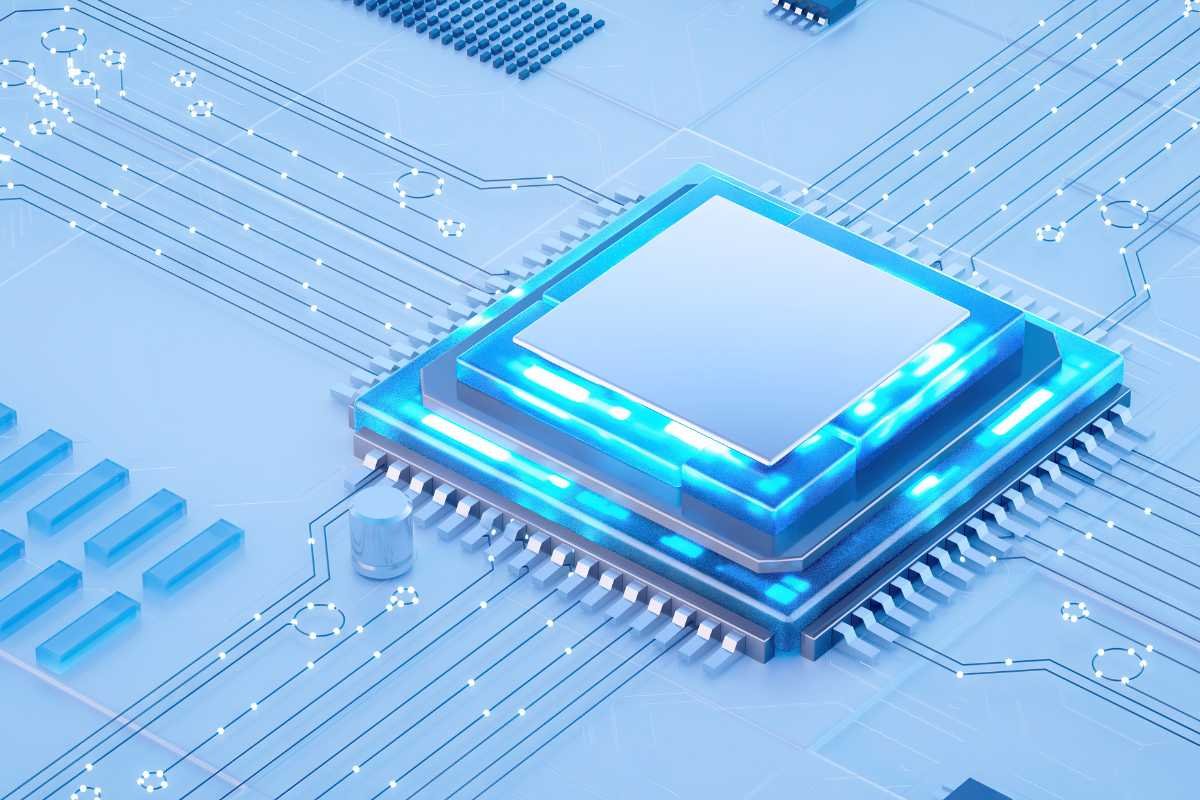
When it comes to AI applications, even in data centers, GPUs take the crown. In data centers, AI is important for performing data analytics. It involves millions of matrix computations. Therefore, data center CPUs are ideal because of their parallel architecture. They have massive computational power that helps speed up data analytic processes.
Datacenter GPUs and CPUs: Strengths
In addition to versatility, CPUs have several other strengths. They include:
- Multi-tasking, which makes them ideal for threaded applications like AI data analytics.
- High speeds, which allows them to complete single-thread tasks at higher speeds.
- When it comes to GPUs, strengths go beyond parallel processing. Other strengths of data center GPUs are:
- High memory bandwidth, which enables them to access and manipulate data faster.
- Energy efficiency, allows them to deliver high performance without consuming as much power as CPUs. This ultimately results in lower data center operating costs.
- Customizability for specific applications, making them adaptable and flexible.
Datacenter GPUs and CPUs: Weaknesses
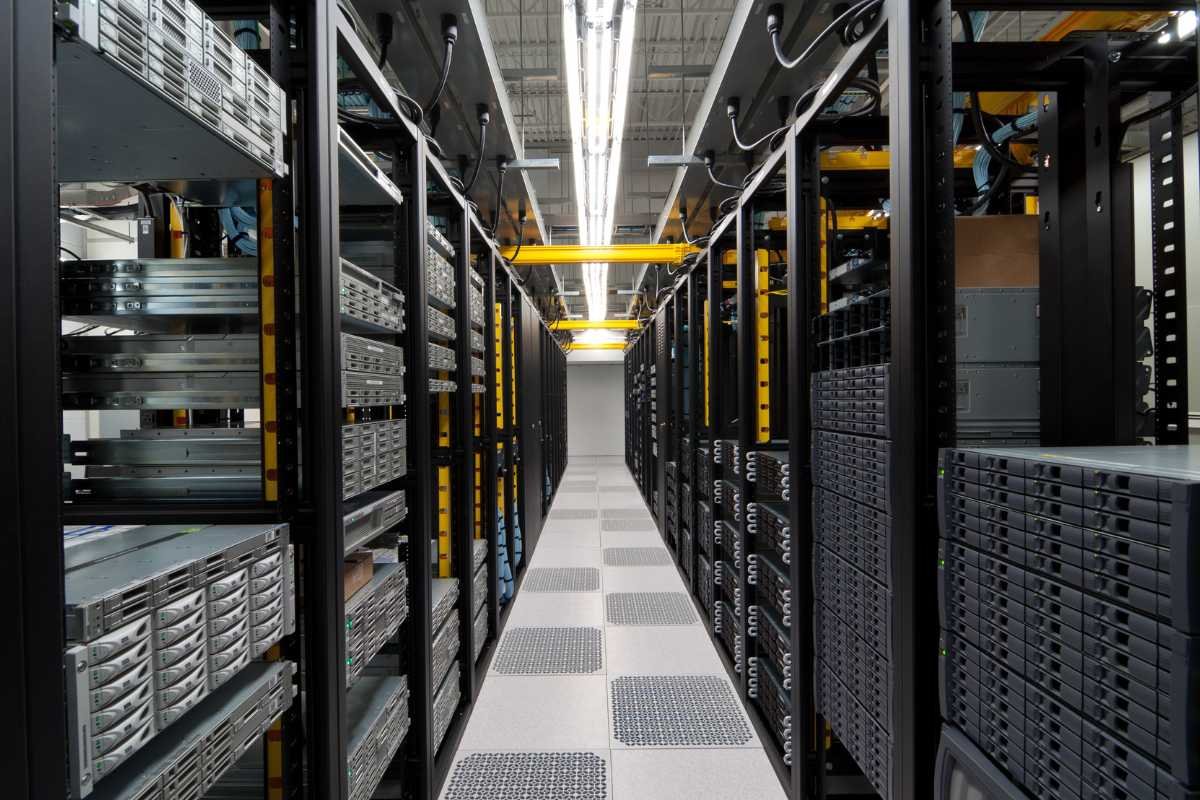
Compared to GPUs, CPUs have limited parallel processing. This means that they cannot perform parallel workloads, which limits their performance in data analytics, especially where AI is involved. so, they consume many energies and have limited memory bandwidth.
On the other hand, compared to CPUs, GPUs are undersigned for concurrent sequential data processing to support the CPUs. They are also notably more expensive to acquire and install and have limited compatibility.
Can GPUs and CPUs work together in data centers?
When handling AI needs in data centers, you have three options. They are:
- CPUs only
- GPUs only
- Both GPUs and CPUs
Most modern computing solutions typically use the capabilities of both GPUs and CPUs for their operations in a heterogeneous computing approach. In this approach, each processor handles different tasks. So, how does this work? It is all about using a different processor for different needs. For example, you can use GPUs to quicken development, training, and data science model refining, while using data center CPUs for handling heavier and more complex model training tasks. Combining the strengths of CPUs and GPUs in data centers enables you to improve performance significantly especially when it comes to scientific simulations and AI applications.
Conclusion
When it comes to data analytics of applications in data centers where AI is used, whether you choose GPUs and CPUs will ultimately depend on your needs. Your budget will also help you make the perfect choice. It is no secret that GPUs are presented as the most suited for AI workloads. However, both processors have their strengths and limitations with CPUs still an important choice in enterprise AI. Understanding this will aid you in making the perfect choice based on your needs.