Clinical trials tend to be complex and data-intensive, which is why efficient data management is crucial. Study data tabulation model (SDTM) datasets play a vital role in this process, providing a standardized format for organizing and reporting clinical trial data.
This guide aims to help you understand how to use SDTM datasets for clinical data integration effectively. Continue reading to learn more!
What are SDTM Datasets?
SDTM datasets are part of the Clinical Data Interchange Standards Consortium (CDISC) suite of standards designed to streamline the collection, organization, and submission of clinical trial data. These datasets provide a standardized structure for presenting clinical trial data in a consistent, reproducible, and analyzable format.
Key Features of SDTM Datasets
A critical aspect of understanding SDTM datasets is knowing their key features. Here are some of them:
1. Standardization
SDTM datasets use a predefined structure and format. This standardization ensures that data from different studies and sources can be easily compared, combined, and analyzed. The use of standard domains and variables facilitates this uniformity.
2. Regulatory Compliance
SDTM datasets comply with regulatory agency requirements, which is why submitting data in SDTM format is often mandatory for the regulatory approval of new drugs and medical devices.
3. Data Consistency
The standard format of SDTM datasets promotes consistency in data collection, reporting, and analysis. This consistency is crucial for accurate data interpretation and minimizes errors and discrepancies.
4. Efficiency in Data Management
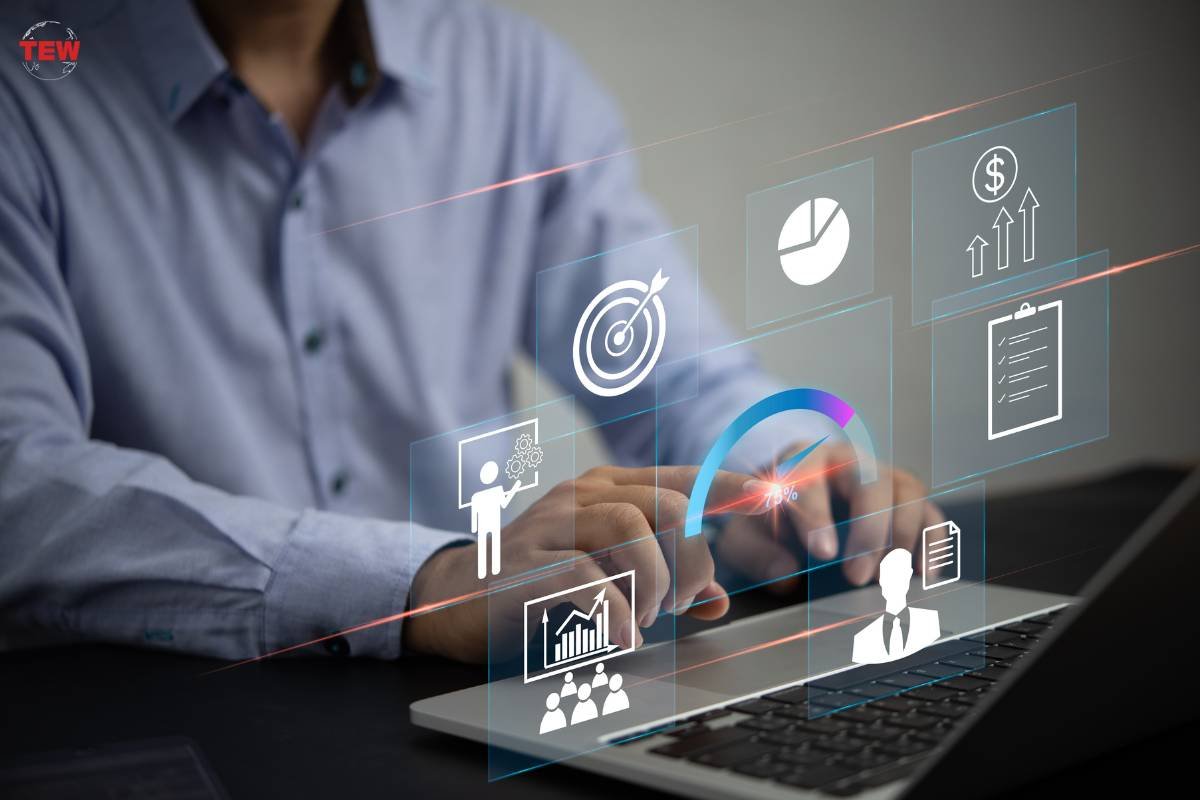
SDTM datasets simplify the data management process by using a standard format. This efficiency is particularly beneficial for large-scale clinical trials, where managing vast data can be challenging.
5. Enhanced Data Integration
SDTM datasets make it easier to integrate data from multiple studies or sources. The common framework allows for seamless merging and comparison of datasets, enhancing the robustness and generalizability of research findings.
6. Improved Data Quality
The standardization and predefined variables reduce the likelihood of errors and inconsistencies in the data. This leads to higher-quality data, essential for making accurate and reliable scientific conclusions.
7. Comprehensive Data Coverage
SDTM datasets cover a wide range of domains, each representing specific aspects of the clinical trial. Common domains include:
- DM (Demographics): Contains demographic information about the study participants
- AE (Adverse Events): Records information about adverse events experienced by participants
- CM (Concomitant Medications): Lists medications taken by participants during the trial
- VS (Vital Signs): Includes measurements of vital signs like blood pressure and heart rate
Each domain has a predefined set of variables, ensuring a consistent format across different studies. Understanding this is crucial for effectively integrating and analyzing clinical trial data.
8. Flexibility for Customization
While SDTM provides a standardized framework, it allows customization to meet specific study needs. Researchers can extend the model with custom domains and variables as necessary, provided they adhere to the overall SDTM structure.
9. Interoperability
The standardized nature of SDTM datasets facilitates interoperability so data can be easily shared and used by different stakeholders, including researchers, clinicians, and regulatory bodies. This interoperability enhances collaboration and data reusability.
SDTM datasets are a cornerstone of modern clinical research, offering a range of features that enhance data management, integration, and analysis. They’re critical to supporting medical research and ensuring the successful development and approval of new therapies.
How to Use SDTM Datasets for Data Integration?
Integrating data from multiple clinical studies or sources is critical in clinical research, and SDTM datasets for clinical data Integration provide a standardized framework that facilitates this process.
Here’s a step-by-step guide on how to use SDTM Datasets for Clinical Data Integration:
1. Data Mapping
Begin by mapping your raw clinical data to the appropriate SDTM domains. Identify the variables in your raw data corresponding to the predefined variables in each domain. This ensures that all necessary data points are captured and organized correctly.
2. Data Transformation
Transform the raw data to fit the SDTM format. This may involve converting units of measurement, standardizing terminologies, and ensuring that all required variables are populated. Use controlled terminology and code lists CDISC provides to maintain consistency across datasets.
3. Data Validation
Validate the transformed data to ensure accuracy and completeness. Check for discrepancies, missing values, and other data integrity issues. Utilize tools and software supporting SDTM datasets to automate and streamline the validation process.
4. Data Integration
Data integration involves merging datasets, aligning variables, and ensuring consistency across the integrated data. Harmonizing variable names and formats is important to avoid conflicts and ensure a smooth integration process.
5. Data Harmonization
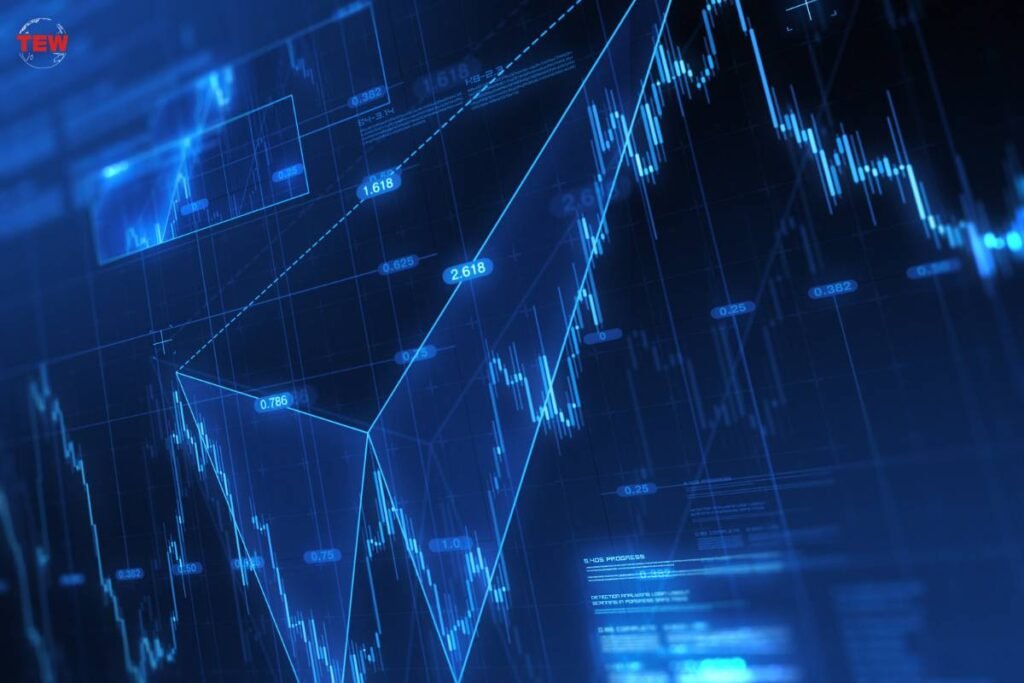
Address any inconsistencies or discrepancies that arise during the integration process. This may include reconciling differences in variable definitions, data formats, or measurement units. Ensure that all integrated data conforms to the SDTM standards, providing a uniform and consistent dataset for analysis.
6. Data Analysis and Reporting
The SDTM datasets for clinical data Integration can now be used for various analyses, such as statistical analysis, safety monitoring, and efficacy assessment. Their standardized format simplifies the analysis process, enabling researchers to generate insights, identify trends, and make data-driven decisions.
7. Regulatory Submission
If the integrated data is to be submitted to regulatory agencies, ensure it meets all regulatory requirements. This includes adhering to the guidelines and submission standards established by agencies like the FDA and EMA. Use submission-ready SDTM datasets to streamline the regulatory review process.
By following these steps and best practices, researchers can ensure that their integrated data is accurate, consistent, and ready for analysis and regulatory submission. This process enhances the quality and reliability of clinical research and supports the successful development and approval of new therapies.
Conclusion
Whether you’re a data manager, biostatistician, or clinical researcher, mastering SDTM datasets is crucial for successful SDTM Datasets for Clinical Data Integration and analysis. Embrace these standards to improve the quality and efficiency of your clinical trials.